NIH issues bias detection tools in health care challenge
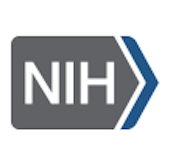
The Minimizing Bias and Maximizing Long-Term Accuracy, Utility and Generalizability of Predictive Algorithms in Health Care Challenge seeks to encourage the development of bias-detection and -correction tools that foster "good algorithmic practice" and mitigate the risk of unwitting bias in clinical decision support algorithms.
Key Dates
Note: Dates subject to change as necessary
- Submission Deadline: March 1, 2023
- Technical Evaluation Phase: March 2023
- Federal Judging Phase: March and April 2023
- Winners Announced: April 2023
- Demo Day: May 5, 2023
Background
Although artificial intelligence (AI) and machine learning (ML) algorithms offer promise for clinical decision support (CDS), their potential has yet to be fully realized. Even well-designed AI/ML algorithms and models can become inaccurate or unreliable over time due to various factors, including changes in data distribution; subtle shifts in the data, real-world interactions and user behavior; and shifts in data capture and management practices. Over time, these changes and shifts can degrade the predictive capabilities of algorithms, which can negate the benefits of these types of systems for clinics.
How do we detect these shifts or changes on a continual basis to maintain prediction quality? Monitoring an algorithm's behavior and flagging any significant changes in performance may enable timely adjustments that ensure a model's predictions remain accurate, fair and unbiased over time. This approach maintains the predictive capability of an algorithm in the real world.
As AI/ML algorithms are increasingly used in health care systems, accuracy, generalizability and avoidance of bias and drift become more important. Bias primarily surfaces in two forms. Predictive bias is seen in algorithmic inaccuracies that produce estimates that significantly differ from the underlying truth. Social bias reflects systemic inequities in care delivery leading to suboptimal health outcomes for certain populations.
To address these issues and improve clinician and patient trust in AI/ML-based CDS tools, this Challenge invites groups to develop bias-detection and -correction tools that foster "good algorithmic practice" and mitigate the risk of unwitting bias in CDS algorithms.
Challenge Goals
The goal of this Challenge is to identify and minimize inadvertent amplification and perpetuation of systemic biases in AI/ML algorithms used as CDS through the development of predictive and social bias-detection and -correction tools. For this Challenge, participants across academia and the private sector are invited to participate in teams, as representatives of an academic or private entity, or in an individual capacity to design a bias-detection and -correction tool.
For most up-to-date information about the rules, submission requirements, judging criteria, prizes, how to enter and to register for the Challenge, please visit the ExpeditionHacks site. You also can visit the Challenge.gov site.
Contact
Have feedback or questions about this challenge? Please send your feedback or question to This email address is being protected from spambots. You need JavaScript enabled to view it..